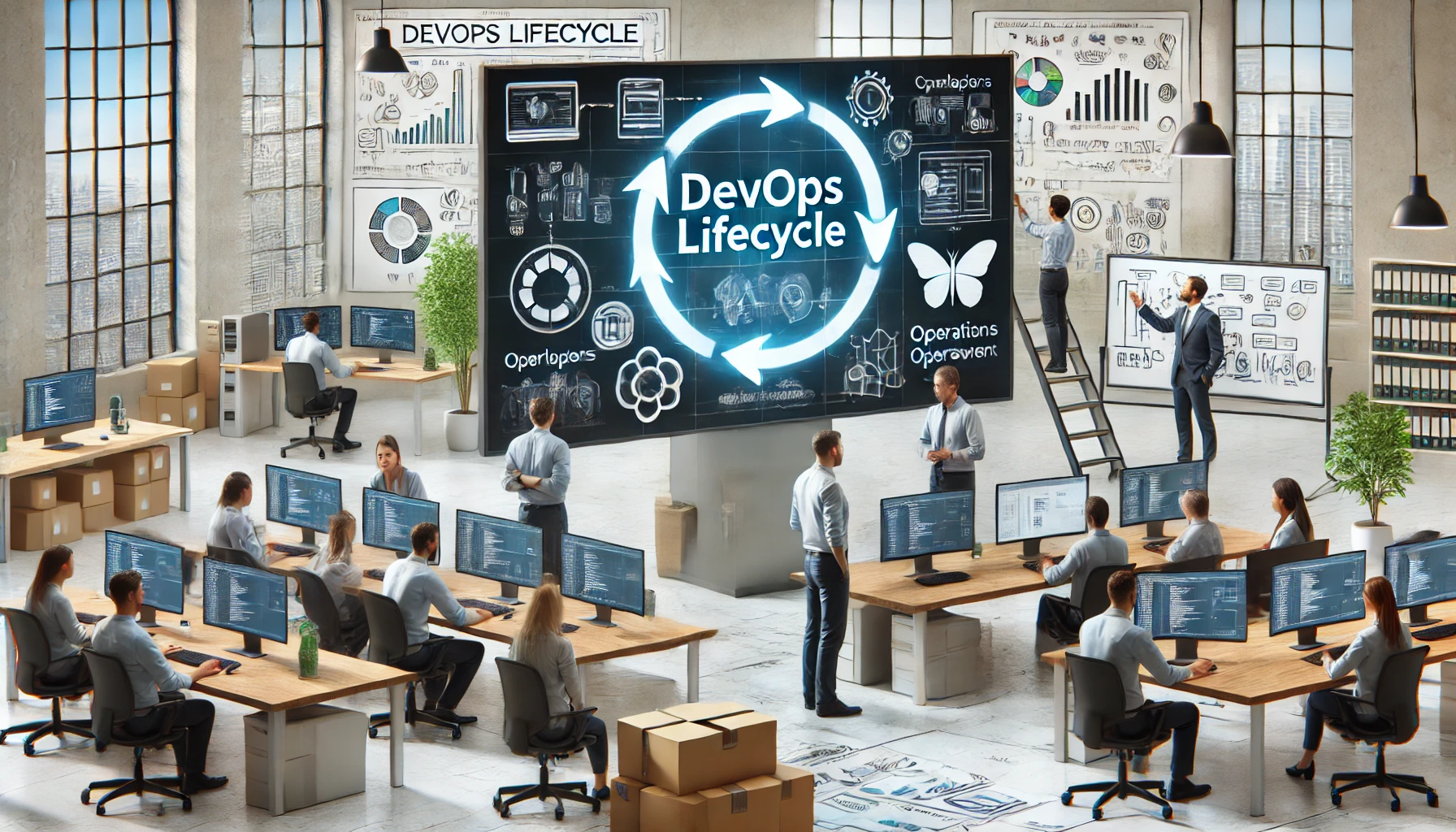
Introduction
In today’s fast-paced software development environment, DevOps has become an essential methodology for delivering high-quality software swiftly. DevOps bridges the gap between development and operations, fostering a culture of collaboration and continuous improvement. This blog post delves into the DevOps lifecycle, highlighting its stages with practical examples and links to additional resources for a deeper understanding.
The DevOps lifecycle is a continuous process composed of several key stages: planning, coding, building, testing, releasing, deploying, operating, and monitoring. Each stage plays a crucial role in ensuring the seamless delivery and maintenance of applications.
Planning
The planning stage involves defining project requirements and setting objectives. Tools like Jira and Trello are commonly used to manage tasks and track progress. For instance, a development team planning a new feature might use Jira to create user stories and tasks, outlining the specific functionality and the steps needed to achieve it.
Additional Material: Atlassian’s Guide to Agile Project Management
Coding
In the coding stage, developers write the application code. Version control systems like Git are used to manage changes and collaborate efficiently. For example, developers working on a new microservice might use GitHub for source code management, ensuring that changes are tracked and can be easily rolled back if necessary.
Additional Material: Pro Git Book
Building
Building involves compiling the source code into executable artifacts. This stage often includes packaging the application for deployment. Using Jenkins for continuous integration, the build process can automatically compile code, run tests, and create Docker images ready for deployment.
Additional Material: Jenkins Documentation
Testing
Automated testing ensures that the application functions correctly and meets the specified requirements. Tools like Selenium and JUnit are popular in this stage. For instance, implementing a suite of automated tests in Selenium to verify the functionality of a web application across different browsers.
Additional Material: SeleniumHQ
Releasing
Releasing is the process of making the application available for deployment. This stage involves versioning and tagging releases. Using Git tags to mark a particular commit as a release candidate, ready for deployment to a staging environment for final verification.
Additional Material: Semantic Versioning
Deploying
Deployment involves moving the application to a live environment. Tools like Kubernetes and Ansible help automate this process, ensuring consistency and reliability. For example, deploying a containerized application to a Kubernetes cluster, using Helm charts to manage the deployment configuration.
Additional Material: Kubernetes Documentation
Operating
In the operating stage, the application runs in the production environment. Ensuring uptime and performance is critical, often managed through infrastructure as code practices. Using Terraform to provision and manage cloud infrastructure, ensuring that environments are consistent and scalable.
Additional Material: Terraform by HashiCorp
Monitoring
Continuous monitoring and logging are essential to detect issues and improve the system. Tools like Prometheus and ELK Stack (Elasticsearch, Logstash, Kibana) are widely used. Implementing Prometheus to collect metrics and Grafana to visualize the performance of a microservices architecture.
Additional Material: Prometheus Documentation
Wrapping it all up
The DevOps lifecycle is a continuous journey of improvement and collaboration. By integrating and automating each stage, teams can deliver robust and reliable software faster and more efficiently. Embracing DevOps practices not only enhances the quality of software but also fosters a culture of continuous learning and adaptation.
For those looking to dive deeper into DevOps, the additional materials provided offer a wealth of knowledge and practical guidance. Embrace the DevOps mindset, and transform your software development process into a well-oiled, efficient machine.
Keep in mind this is a very high level list of some of the most commonly used tools everyday. There’s no mention of platforms here such as Rancher as it was intentionally kept high level. Future content will provide insights into best practices, other platforms, and how to be successful in a Devops world.